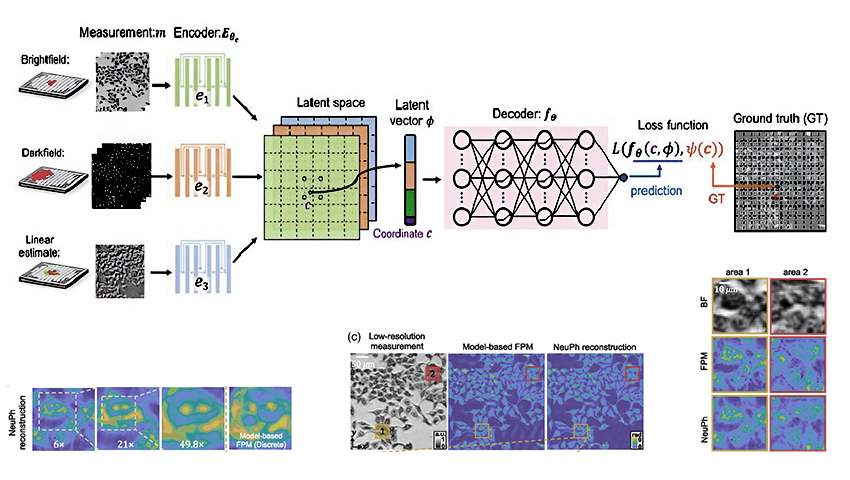
Deep learning continues to push the boundaries of computational imaging, providing advanced solutions to challenges in image reconstruction. A recent innovation, developed by researchers from Boston University’s Computational Imaging Systems Lab, offers a scalable and generalizable neural framework known as NeuPh (Neural Phase Retrieval), which dramatically enhances the reconstruction of high-resolution images from low-resolution data. This novel approach combines advanced neural networks with a deep understanding of physical object structures, allowing for more accurate and reliable image reconstructions.
Historically, image reconstruction methods have relied on discrete pixel representations, limiting the ability to capture the continuous and multiscale nature of real-world objects. These limitations are especially evident in fields such as biomedical imaging, where capturing intricate structures at high resolution is critical. Traditional methods, constrained by the diffraction limit and noise, often struggle to provide sufficient detail. NeuPh addresses this by leveraging deep learning models that can interpret and reconstruct continuous object features from noisy, low-resolution inputs.
At the core of NeuPh is a two-stage neural network architecture. The system first employs a convolutional neural network (CNN) encoder that processes low-resolution images, compressing them into a latent space where key information is represented efficiently. This latent space allows the system to handle complex structures without the need for full high-resolution data input.
The second component is a multilayer perceptron (MLP) decoder, responsible for reconstructing the high-resolution phase information from the latent representation. This approach allows the system to handle multiscale information, offering a more complete and detailed reconstruction than traditional pixel-based models. The result is a high-quality image that captures subtle details and minimizes artifacts such as noise and phase unwrapping errors.
One of the standout features of NeuPh is its ability to generalize across different datasets and experimental conditions. Trained on both simulated and experimental data, the system shows remarkable flexibility, performing well even when data is scarce or imperfect. This generalization capability is particularly important in real-world applications, where training conditions often differ significantly from operational scenarios. NeuPh’s adaptability is further enhanced by its ability to reconstruct images that surpass the diffraction limit of the input measurements, achieving “super-resolution”.
The potential applications of NeuPh are vast. Its ability to deliver high-resolution, artifact-free reconstructions from limited data makes it an ideal candidate for various fields, including biomedical imaging, materials science, and beyond. The combination of deep learning with physical models offers a pathway to more accurate and scalable imaging systems, capable of handling the most complex structures and environments.
Explore more details of the research in the publication in SPIE Digital Library.