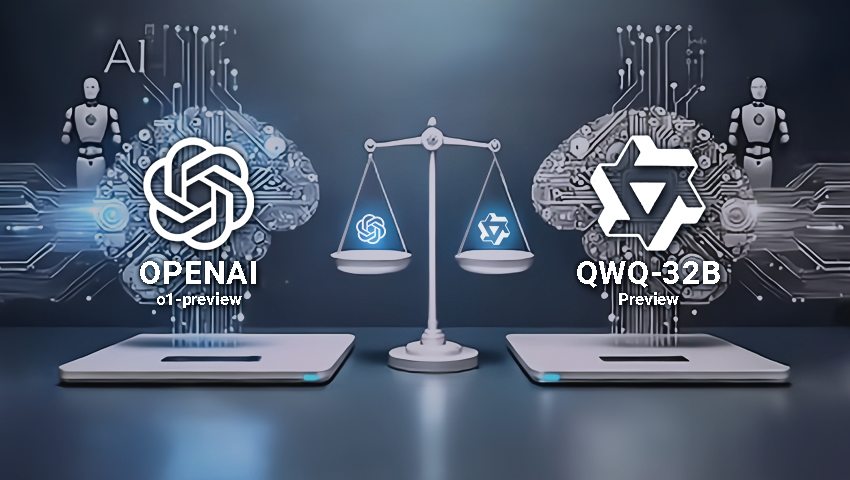
A new AI model, QwQ-32B-Preview, has emerged as a strong contender in the field of reasoning AI, especially as it’s available under an Apache 2.0 license, i.e. open for commercial use. Developed by Alibaba’s Qwen team, this 32.5 billion parameter model can process prompts of up to 32,000 words and has outperformed OpenAI’s o1-preview and o1-mini on certain benchmarks.
According to Alibaba’s testing, QwQ-32B-Preview outperforms OpenAI’s o1-preview model on the AIME and MATH tests. AIME evaluates models using other AI systems, while MATH consists of a collection of challenging word problems. The new model’s reasoning capabilities enable it to tackle logic puzzles and solve moderately difficult math problems, though it is not without limitations. For instance, Alibaba has acknowledged that the model can unexpectedly switch languages, become trapped in repetitive loops, or struggle with tasks requiring strong common-sense reasoning.
Unlike many traditional AI systems, QwQ-32B-Preview includes a form of self-checking mechanism that helps it avoid common errors. While this approach enhances accuracy, it also increases the time required to produce solutions. Similar to OpenAI’s o1 models, QwQ-32B-Preview employs a systematic reasoning process, planning its steps and executing them methodically to derive answers.
QwQ-32B-Preview is accessible on the Hugging Face platform, where it can be downloaded and used. The model’s approach to sensitive topics aligns with other reasoning models like the recently released DeepSeek, both of which are influenced by Chinese regulatory frameworks. As companies like Alibaba and DeepSeek operate under China’s stringent internet regulations, their AI systems are designed to adhere to guidelines that promote “core socialist values.” This has implications for how the models respond to politically sensitive queries. For example, when asked about Taiwan’s status, QwQ-32B-Preview provided an answer consistent with the Chinese government’s stance. Similarly, prompts about Tiananmen Square resulted in non-responses, reflecting the regulatory environment in which these systems are developed.
While QwQ-32B-Preview is marketed as available under permissible license, not all components of the model have been released. This partial openness limits the ability to replicate the model fully or gain a comprehensive understanding of its architecture. The debate over what constitutes “openness” in AI development continues, with models ranging from entirely closed systems, offering only API access, to fully open systems that disclose all details, including weights and data. QwQ-32B-Preview occupies a middle ground on this spectrum.
The rise of reasoning models like QwQ-32B-Preview comes at a time when traditional AI “scaling laws” are being questioned. For years, these laws suggested that increasing data and computing resources would lead to continual improvements in AI capabilities. However, recent reports indicate that the rate of progress for models from leading AI labs, including OpenAI, Google, and Anthropic, has begun to plateau. This has spurred a search for innovative approaches in AI development, including new architectures and techniques.
One such approach gaining traction is test-time compute, also known as inference compute. This method allows AI models to use additional processing time during tasks, enhancing their ability to handle complex challenges. Test-time compute forms the foundation of models like o1 and QwQ-32B-Preview, reflecting a shift in focus toward optimizing performance during inference rather than solely relying on training.
Major AI laboratories beyond OpenAI and Chinese firms are also investing heavily in reasoning models and test-time compute. A recent report highlighted that Google has significantly expanded its team dedicated to reasoning models, growing it to approximately 200 members. Alongside this expansion, the company has allocated substantial computing resources to advance this area of AI research, signaling the industry’s growing commitment to the future of reasoning AI.