Diffusion, FM & Pre-Trained AI models for Time-Series. DeepNN-based models are starting to match or even outperform statistical time-series analysis & forecasting methods in some scenarios. Yet, DeepNN-based models for time-series suffer from 4 key issues: 1) complex architecture 2) enormous amount of time required for training 3) high inference costs, and 4) poor context sensitivity.
Latest innovative approaches. To address those issues, a new breed of foundation or pre-trained AI models for time-series is emerging. Some of these new AI models use hybrid approaches borrowing from NLP, vision/ image, or physics modelling, like: transformers, diffusion models, KANs and state space models. Despite the scepticism in the hard-core statistics community, some of these new AI models for time-series are producing some surprisingly good results. Let’s see…
Foundation Models for Time-series. This new survey examines the effectiveness, efficiency and explainability (3Es) of pre-training foundation models from scratch for time series, and adapting large language foundation models for time series. The survey comes with an accompanying repo loaded with awesome papers, code and resources. Paper, repo: A Survey of Time Series Foundation Models & Awesome-Time Series-LLM&FM.
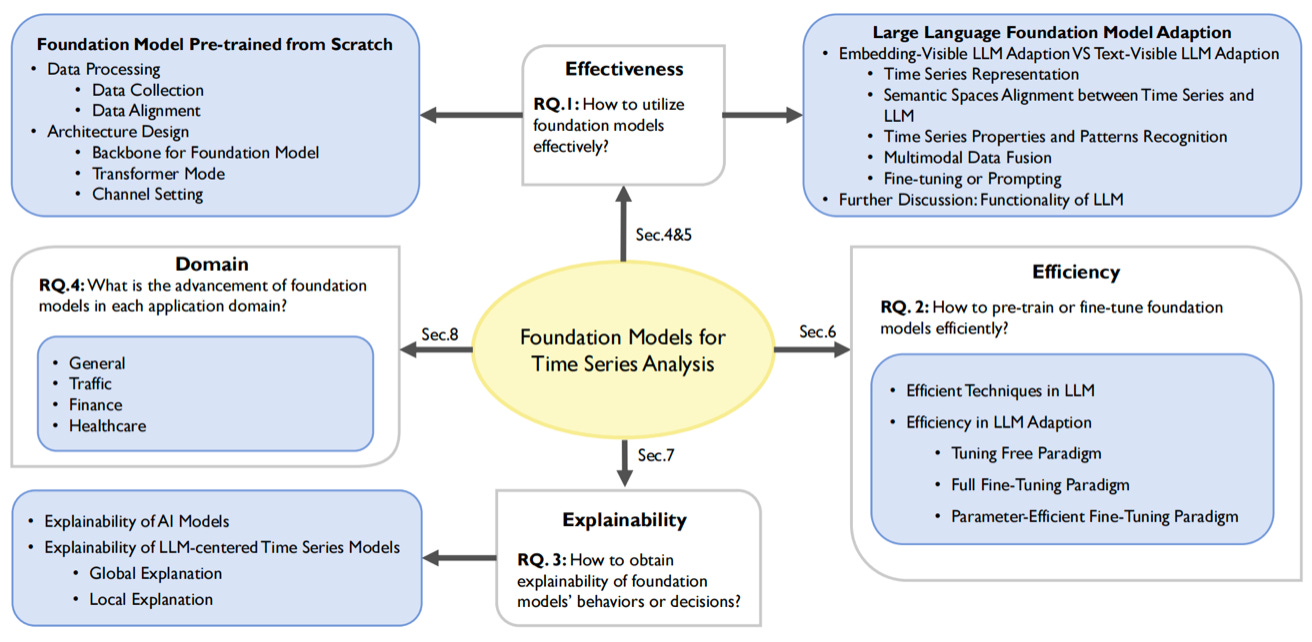
Diffusion Models for Time-Series. A recent, comprehensive survey and systematic summary of the latest advances in Diffusion Models for time series, spatiotemporal data and tabular data with more awesome resources (paper, code, application, review, survey, etc.). The paper also provides a great taxonomy of diffusion models for time series. Paper, repo: Diffusion Model for Time Series and Spatio-Temporal Data.
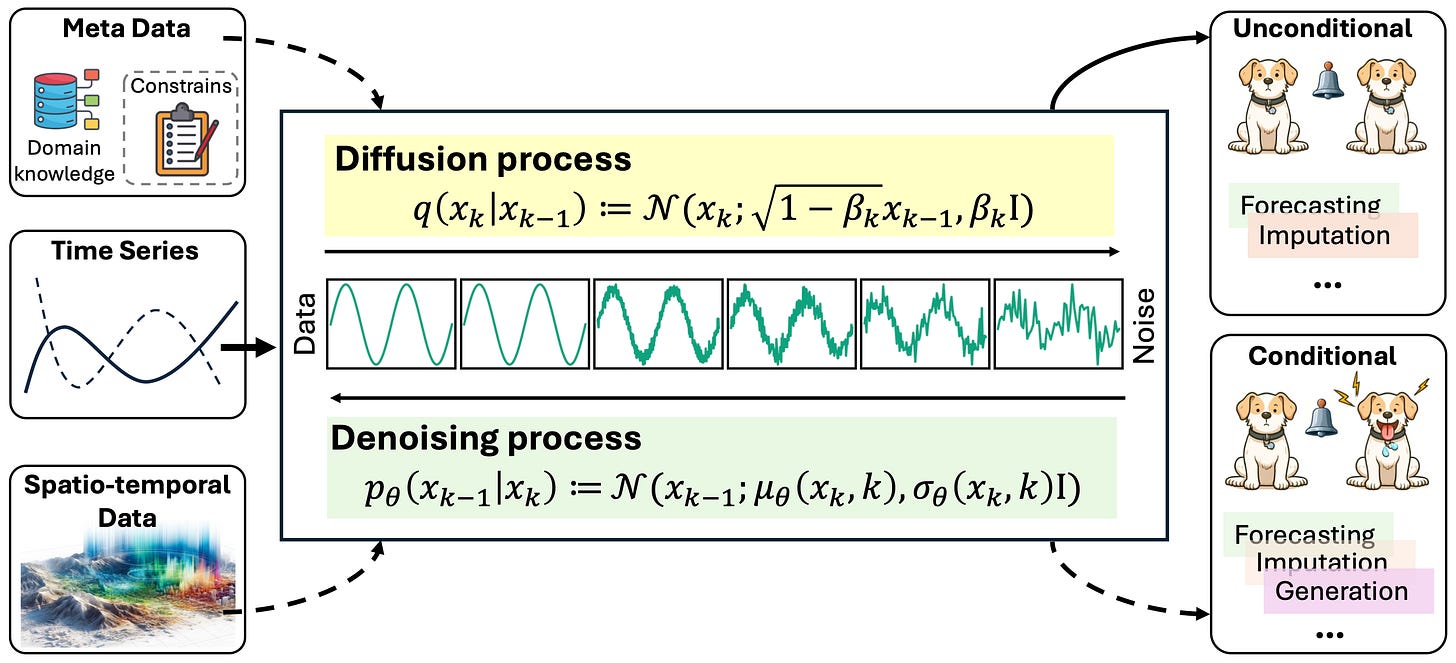
TinyTimeMixers (TTMs). A new family of compact pre-trained models for Multivariate Time-Series Forecasting, open-sourced by IBM Research. With less than 1 Million parameters, TTM introduces the notion of the first-ever “tiny” pre-trained models for Time-Series Forecasting. Checkout the TTMs model card, paper and getting started notebook here.
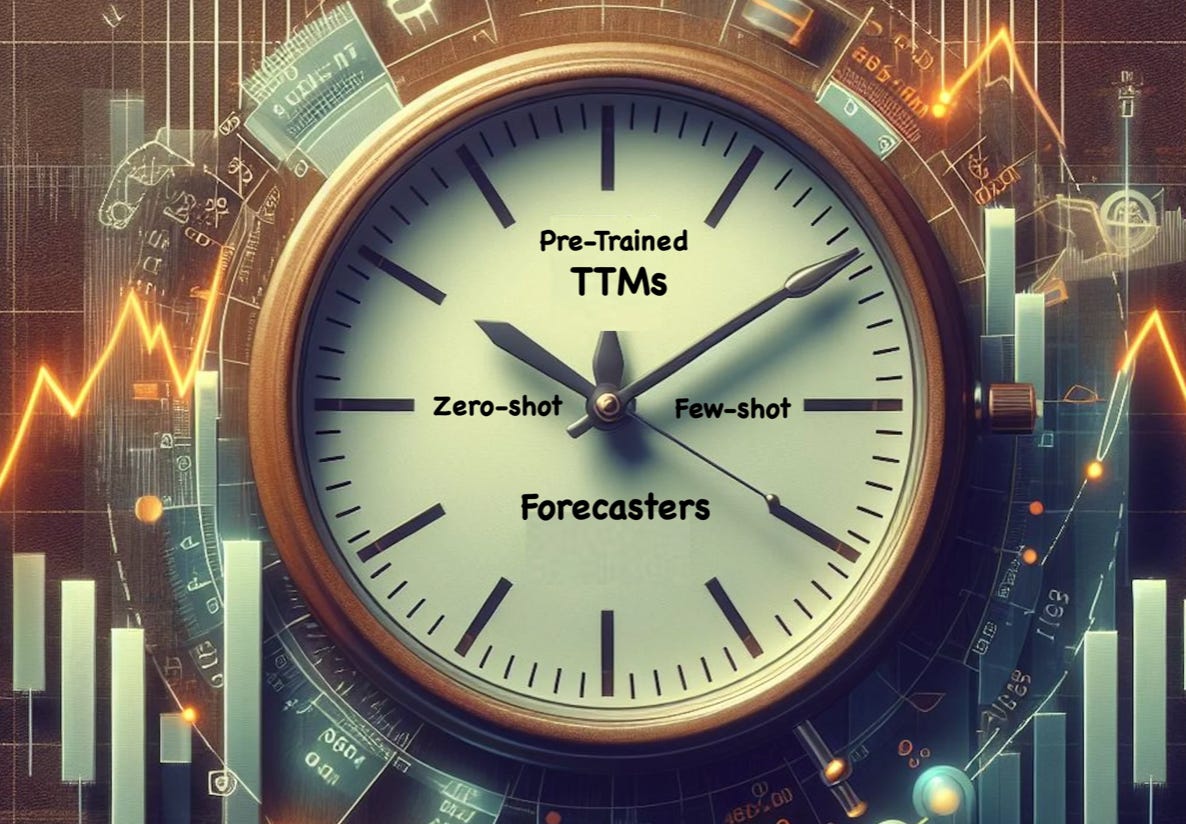
MambaFormer for Time-series. MambaFormer is a new hybrid model that for the first time combines Mamba (a state space model) for long-range dependency, and the Transformer for short range dependency, for long-short range forecasting. The researchers claim the MambaFormer outperforms both Mamba and Transformer in long-short range time series forecasting. Paper: Integrating Mamba and Transformer for Long-Short Range Time Series Forecasting.
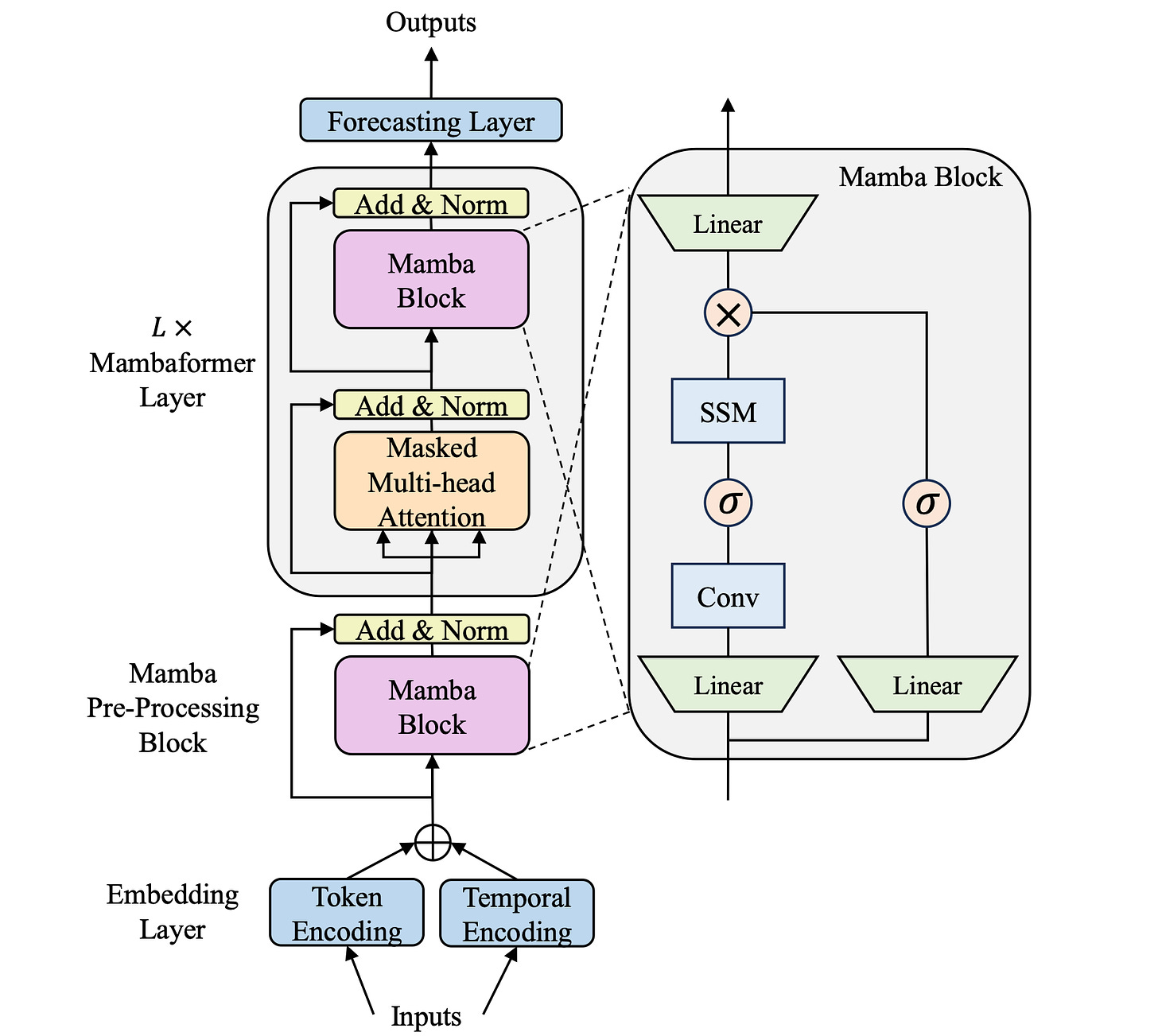
Google TimesFM is a new forecasting model, pre-trained on a large time-series corpus of 100 billion real world time-points, that displays impressive zero-shot performance on a variety of public benchmarks from different domains and granularities. Paper, repo: A decoder-only foundation model for time-series forecasting.
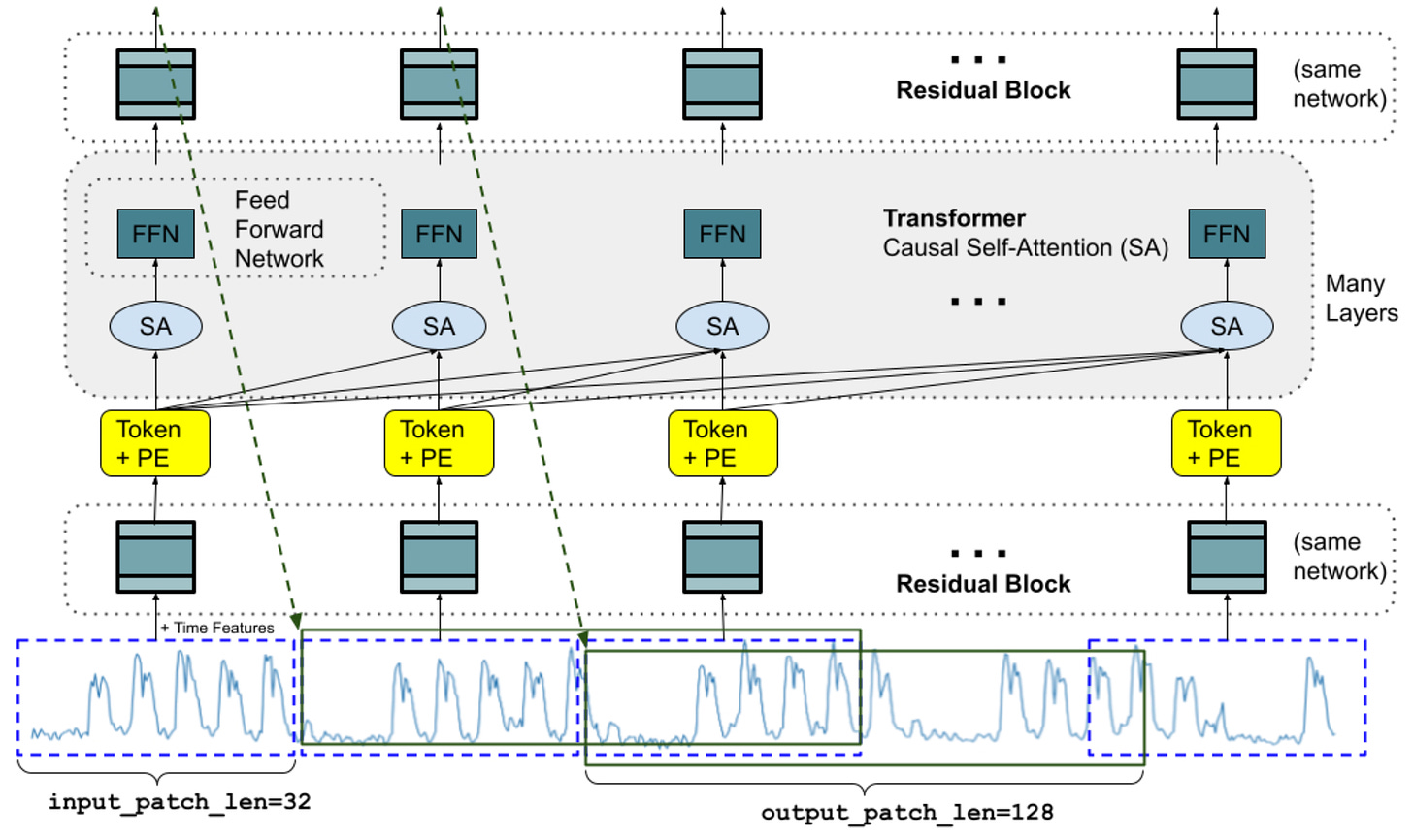
DeepKAN. This is a very new approach that combines a neural network with Kolmogorov-Arnold Networks (see link #7 below on KANs.) The researchers say that KANs outperform MLP (Multi-Layer Perceptron)-based methods in time-series prediction tasks when applied to the Air Passengers dataset (although not a very exciting/complex dataset.) DeepKAN: Deep Kolmogorov-Arnold-Networks-KAN.
Have a nice week.
-
BabyAGI, AI Agents & AI Investing
-
Avoiding the Frankenstein Prompt
-
The ICLR 2024 Outstanding Paper Awards
-
How to Create AI Agents that Don’t Suck
-
Seven Low-Hanging Fruits for RAG Search
-
What is Context Attribution in Generative AI?
-
Understanding Kolmogorov–Arnold Networks (KANs)
-
A Review of S/W Engineering (SWE) AI Agent Tech Report
-
A New SOTA Model for Data Labelling, Enrich. & Cleaning
-
Why I’m Building My Own AI Agent Library (blogpost & repo)
-
ScrapeGraphAI: You Only Scrape Once with AI (repo, demo)
-
Create LLM Agents with Long-term Memory and Custom Tools
-
GPT Researcher – An Autonomous Agent for Online Research (repo, demo)
-
[free hands-on] Deep Reinforcement Learning: Zero to Hero!
-
Tackling Vanishing and Exploding Gradients in DeepNNs
-
[free course] UT Austin CS388- Modern NLP, Masters-level
-
xLSTM: Extended LSTM by Leveraging Transformers Concept
-
Google AI – Leverage Foundational Models for Black-Box Optimisation
-
MS Research – Visualization-of-Thought Elicits Spatial Reasoning in LLMs
-
Building ML Models Through Automated Augmented Tuning
-
[review] 7 Best ML Workflow & Pipeline Orchestration Tools
-
How to Deploy an End-to-End Private RAG App on Kubernetes
-
Lichess Puzzles – A Collection of ~1.5M Chess Puzzles
-
Buzz – An Instruction-Following Dataset, 85 Million Conversations
-
Google ImageInWords: Hyper-Detailed Image Descriptions & Annotations
Tips? Suggestions? Feedback? email Carlos
Curated by @ds_ldn in the middle of the night.