Drug-induced toxicity is a major challenge in drug development, contributing significantly to the failure of clinical trials. While efficacy issues account for most failures, safety concerns are the second leading cause, at 24%. Toxicities can affect various organ systems, including the heart, liver, kidneys, and lungs, and even approved drugs may face withdrawal due to unforeseen toxic effects in post-market surveillance. Current toxicity datasets, often derived from labor-intensive expert analyses of FDA drug labels, are typically small and limited to specific organ systems. These documents, which detail a drug’s indications, risks, and clinical trial results, are critical but time-consuming to curate, often exceeding 100 pages per drug. Consequently, there is a pressing need for predictive models to identify safer drug candidates early in development.
Efforts to build comprehensive toxicity datasets have faced several limitations. Existing databases, such as SIDER, LiverTox, and PNEUMOTOX, are often organ-specific or rely on in vitro assays, which may not accurately predict in vivo effects. Annotation efforts are time-intensive, and methodologies for toxicity evaluation vary widely, leading to inconsistencies across datasets. For instance, the FDA’s renal toxicity database, DIRIL, integrates conflicting sources with over 30% disagreement on certain drugs. Large language models (LLMs) like askFDALabel offer promise by streamlining data extraction from FDA labels, achieving up to 78% agreement with human evaluations for cardiotoxicity. However, despite advancements, challenges in dataset scalability, annotation consistency, and comprehensive coverage persist, limiting the effectiveness of ML models trained on these datasets.
Researchers from Stanford University and Genmab introduced UniTox, a comprehensive dataset of 2,418 FDA-approved drugs, summarizing and rating drug-induced toxicities using GPT-4o to process FDA drug labels. Covering eight toxicity types, including cardiotoxicity, liver toxicity, and infertility, UniTox is the largest systematic in vivo database and the first to encompass nearly all non-combination FDA-approved drugs for these toxicities. Clinicians validated a subset of GPT-4o annotations, with concordance rates of 85–96%. Benchmarks of machine learning models trained on UniTox demonstrated its utility for predicting molecular toxicity, achieving up to 93% accuracy on existing datasets and surpassing askFDALabel’s performance.
To develop UniTox, researchers curated a dataset of 2,418 FDA-approved drugs by filtering and deduplicating drug labels from the FDALabel database, including biologics. Using GPT-4o and a two-step chain-of-thought prompting system, the team generated toxicity summaries and ratings for eight toxicity types. The model categorized toxicity using ternary (No, Less, Most) and binary (Yes, No) scales. The validation included comparisons with existing FDA datasets (DICTrank, DILIrank, DIRIL) and clinician reviews, achieving strong concordance. Clinicians evaluated a subset for toxicity types lacking prior data, scoring the model’s outputs based on factual accuracy and alignment with expert knowledge.
The UniTox dataset, encompassing 2,418 drugs and eight toxicity types, offers a comprehensive resource for toxicity analysis. It includes GPT-4o-generated toxicity summaries, ternary and binary classifications, and Structured Product Labeling (SPL) IDs. Summaries condense lengthy drug labels into 297 words on average, aiding quick comprehension and enabling their use as ground truth for training toxicity predictors. The dataset reveals toxicity correlations, with liver and hematological toxicity showing the highest relationship (0.45). UniTox also provides insights into toxicity patterns across drug classes based on WHO-ATC classifications, highlighting variations linked to FDA risk tolerance for different therapeutic categories.
In conclusion, the study highlights the use of GPT-4o for efficiently summarizing complex drug labels, producing accurate toxicity ratings across eight types, including liver, renal, and cardiotoxicity. These ratings showed strong agreement with datasets like DILIrank and clinical reviewers, enabling training molecular classifiers with predictive value. The UniTox dataset, comprising 2,418 FDA-approved drugs, is the largest and bridges gaps in toxicity evaluation across multiple organ systems. Despite challenges like nuanced toxicity translation and limited applicability to failed drugs, UniTox demonstrates the value of LLMs in creating detailed datasets, advancing drug toxicity prediction, and supporting future research efforts.
Check out the Paper and Dataset. All credit for this research goes to the researchers of this project. Also, don’t forget to follow us on Twitter and join our Telegram Channel and LinkedIn Group. Don’t Forget to join our 60k+ ML SubReddit.
🚨 Trending: LG AI Research Releases EXAONE 3.5: Three Open-Source Bilingual Frontier AI-level Models Delivering Unmatched Instruction Following and Long Context Understanding for Global Leadership in Generative AI Excellence….
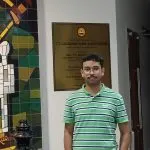
Sana Hassan, a consulting intern at Marktechpost and dual-degree student at IIT Madras, is passionate about applying technology and AI to address real-world challenges. With a keen interest in solving practical problems, he brings a fresh perspective to the intersection of AI and real-life solutions.